EP30: ChatGPT Enterprise, Are Wrapper Apps Doomed? Prompt2Model & Synthetic Training Data.
Speaker 1 (00:00:01):
When you think of the world's biggest tech hubs, you think of Hong Kong, Japan, Silicon Valley, but I'm on my way to a sleepy fishing town on the Cornish coast, a good five to six hours south of London to not just meet but actually have a conversation with the world's most advanced human-like robot. I'm here to help you learn. Shut up Amika. On this day Will is her master and theirs is definitely an old fashioned relationship.
Speaker 2 (00:00:30):
Amika shut up
Michael Sharkey (00:00:34):
To Chris. The big announcement this week was that open AI's chat, G P T has gone enterprise.
Chris Sharkey (00:00:42):
Yeah, exactly. It had to happen, right?
Michael Sharkey (00:00:44):
Yeah, and so they've released enterprise grade security and privacy into chat G P T and that basically, I think to summarise it just means that they're not going to use your data to train on, which is what everyone wanted.
Chris Sharkey (00:00:59):
A bit like, oh, you wouldn't want someone to come and smash the glass of your store, would you better pay us?
Michael Sharkey (00:01:06):
Yeah. Well this was one of the reasons why no one or a lot of enterprises were actually banning chat G P T in the first place. They were worried obviously about proprietary data being shared, so they've addressed that with chat G P T Enterprise. I thought probably the most interesting parts of it were they say unlimited access to G P T four, no usage caps, higher speed performance. We assume that's because it's going to be hosted on Azure two times faster
Chris Sharkey (00:01:32):
And 32 K prompts as
Michael Sharkey (00:01:34):
Well. Yeah, and the big 1 32 K prompts. The other thing is shareable chat templates, but I did see a leak screenshot of this today, what it actually looks like and it's not that exciting. It's just literally what you would think about. It's like a text box and you can put in a prompt and share it with others. They
Chris Sharkey (00:01:53):
Definitely seem to me, as far as web application development goes, they're either not prioritising it or they're amateurs at it because you could add a lot of really, really simple commodity web app stuff to make their app a lot better, but they just don't seem to, it seems like a sort of V one very early V one.
Michael Sharkey (00:02:13):
Yeah, I guess it comes back to the question of they just making so much money that they feel that they can and they're so far ahead that they can just slowly iterate and add incremental features, but the other call outs that they set at the end of this story is we've got more features in the works and we'll launch them as soon as they're ready. Customization, securely extend chat, GT's knowledge with your company data by connecting the applications you already use. That sort of sounds to me like embeddings or maybe allowing you to connect to your data warehouse or different data sources and then they had availability for all team sizes, so this is something that a lot of people online have called out right now. You've got to talk to sales and have a big enough team to warrant buying into chat GT enterprise, so they're talking about a self-serve chat GT business offering for smaller teams, so in theory, other teams would be able to access this if you've got a four or five person company, they're talking about additional power tools for what they call advanced data analysis now, which is just code interpreter renamed.
(00:03:20):
Everyone's criticising how they've named that and it's because it is absolutely shocking. Advanced data analyst.
Chris Sharkey (00:03:27):
It definitely seems like, yeah, they're going into the application space, which is a little confusing and it seems a little bit like a company that doesn't really know what it wants to be.
Michael Sharkey (00:03:38):
Yeah, we were talking about this before recording in the sense that this is a full-blown move into enterprise software and releasing enterprise features, all the compliance that comes with that, and then the last point I'll call out before we move on is solutions for your function. More tools for specific roles such as data analysts, marketers, customer support, and more. So they're clearly developing custom business and enterprise solutions here with enterprise software, but their nominated goal is leading this path to a G I, so I just don't get it. It's like you becoming a B two B SaaS enterprise company built on top of your APIs or are you trying to be a build the best models and yeah,
Chris Sharkey (00:04:25):
It's like vertical integration gone mad or something. They're not quite sure at what level they want to operate and I do find that confusing because what we've always said is that we actually think the future of the deployment of models is going to be within other applications and directly integrated into the tools you normally use or new tools that will come out rather than you go to some AI dedicated application and copy and paste or integrate your data into it and then take that out back into whatever you were doing. They're sort of on the inconvenience side of using it.
Michael Sharkey (00:04:59):
Yeah, that is the point I've made often is that these tools, if you look at what Microsoft is trying to do with being enterprise, which is essentially the same thing in a way, and then how they're embedding it into all of their office tools. Do people that are just not up to speed with this kind of stuff or do they just go and use the Microsoft tools or do they go and use the Google tools because that's built into their existing workspaces and connected to their corporate network and their corporate data? It just seems like that's probably the way it pans out as opposed to you having this separate application and then having to wire all the data into chat G B T.
Chris Sharkey (00:05:43):
Yeah. I wonder if part of it though is them just protecting their revenue in the short term, so they're really keen on the A G I stuff. They're keen on building the newest, greatest models, but they can't have these 20% drops in revenue. I mean I know we're going to talk about them having huge revenue, so it might not be that, but perhaps it's just look, if we announce enterprise grade security and enterprise tools, companies will continue to use us on mass, so we'll keep our revenue up while we pursue these other goals that we actually care about sort of Elon Musk, how he's like, oh, I just built all this crap so I can get money to go to Mars.
Michael Sharkey (00:06:15):
Yeah, that's a pretty good point. Maybe it's just building these tools to fund the mothership so they can keep marching towards their mission, which is a pretty good goal, but then you've got all of the people that are paying the bills, I think paying probably the most of the bills right through the APIs like say Salesforce that announced a series of AI features I think last week or the week before where they've really got all of your data or enterprise data stored in the cloud already and they're just building these experiences into your already existing workflows and then I just don't really understand how this aligns with them being a customer of OpenAI, but then you've got chat G B T enterprise coming out in competition to that as well.
Chris Sharkey (00:07:07):
Yeah, I just dunno the answer. You're right. It is indirect competition to all of the things that they're promoting that you can use their a p I for. They're like, we'll also do it and we'll give you templates that you can share so you can do it too.
Michael Sharkey (00:07:20):
The real question about this product to me is, is this just a niche product? Things like what are they calling it now? I already forget, data analyzer or something. Those tools are not necessarily that easy to use right now. You've got to know the scope of them, you've got to play around with them a lot to get familiar with what you can actually do and I'm just not sure I can see this kind of technology spreading wide in the enterprise when right now it's just a lot of enthusiasts and people playing around with it to figure different things
Chris Sharkey (00:07:54):
Out. Yeah, I agree with that. I think the thing about the use of the modern large language models is there are two very distinct kinds of usage. There's the casual experimental style usage, which is how I'd categorise using it for a school assignment, using it to write a document for your work and things where it's like zero shot or multi-shot use of the large language model to just do whatever writing code, all that basic stuff. But then there's the fully committed, we're going to customise a model, we're going to train data, we're going to write detailed fixed prompts and test them and actually investing time and money as a company. And I would argue at the moment, if you're going down the second path, then you'd be much better to use an open source model like LAMA two and fine tune it for your own purposes. Then you would going off and paying for commercial tools when it's actually more difficult to do it even through their new tools than it would be to do it yourself.
Michael Sharkey (00:08:52):
Yeah, I guess is as they bring out bigger context windows and I'm assuming they'll have some sort of embedding solution in the future, which is what they allude to. A company's just willing to pay a subscription service to open AI forever or indefinitely and to host their train models on their end, use that application because they don't have the expertise to go and train their own models on open source and build interfaces and do all of these various things. So I don't know. It generally feels like in these kind of categories it really is somewhat of a winner takes all approach. If they get this into the enterprise first, then do they just win because that's what everyone's default uses? Yeah,
Chris Sharkey (00:09:37):
I think it'll deeply depend on how well their fine-tuning interface is. I assume it's going to be fine tuning when it comes to that data or like you say embedding or something, but right now those things are very sparsely documented, A P I docs if you want to use them, and so they're going to have to go a long way in terms of their application. This is an application, remember when they just added the function stuff, which doesn't even always work three weeks ago. They're not exactly blowing people away with their ability to build enterprise SaaS software, so they're really going to have to step up their game if people are going to invest at that level to have their stuff on there. I get what you're saying. Some companies are not going to have the expertise to do it themselves. Most won't. So if there is something that does it for you, then great, but they're really just not demonstrating at the moment that they have the expertise on that side of application development.
Michael Sharkey (00:10:31):
I also found it interesting for a company that's really built on the back of self-service where you just go use the product, it's amazing, you want to use it more so you put in your credit card, pay the $20 a month and get greater access to it and people have put up, as you say, with a lot of bugs where they were sharing chat histories with other users accidentally and it going down all the time while they were paying customers and
Chris Sharkey (00:10:58):
Removing features like the web browse thing for example,
Michael Sharkey (00:11:02):
And people have put up with a lot and then to turn around and have contact sales for your enterprise product, it just feels a little bit odd now you've got a sales team, you're adding this complexity to the business. I think what you said earlier is what are they trying to be here If they announce we're just going fully after business and enterprise with this technology and building out solutions and use cases for them, it would be very logical to go and build all these teams and various components of the business and focus your energy solely on that. But I always worry in the early stages of an organisation when they're trying to focus on going and building this sort of enterprise SaaS offering essentially, and then also, oh, we're going to build models to solve a G I when there's all this increased competition or seemingly increasing competition out there from open source models.
Chris Sharkey (00:11:59):
Yeah, exactly. You can't be the best at both and they're definitely going to be mediocre at one. Clearly right now they're building the best models, so I just don't see them being the enterprise winner. And I know you keep saying you think it's sort of winner takes all, but I think the market is so fragmented now. I just don't see how one company can dominate. Maybe they can for a while, but I just don't see how it is a winner takes all scenario here. Yeah,
Michael Sharkey (00:12:28):
It's still remains to be seen whether people just use AI in the context of their day-to-day workflow or they do want to go to something like chat G B T and have this other relationship or other app in that stack that's just connecting in well to all these elements. I'd be curious, especially for our listeners who can comment on YouTube, if you're using chat G B T in the workplace, would you be interested in getting the enterprise edition or this self-serve business edition? And if you are, why and what are you using it for? I'd be curious just to hear from you to see how people either intend to use this or are they just not that interested in it as a product?
Chris Sharkey (00:13:07):
Yeah, I think the SOC two certification is really significant because it'll mean it can come back into businesses that have banned it. So I think that it'll definitely help them in terms of getting people back to it who've decided as organisations that it's to be banned because now with that kind of security and guarantee that the data is safe, that I think that more people will use it Again, I just don't see it as particularly sustainable when there's going to be other point solutions as part of existing products and also just dedicated enterprise products that solve specific problems rather than a generalist.
Michael Sharkey (00:13:42):
I also wonder if there's scar tissue from the whole Facebook era of the last sort of era of social media development where these companies didn't take privacy very seriously at all. I mean Facebook is known for laughing about how people gave it data and they could see it all. I just wonder if the enterprise is scarred from these tech companies controlling their access to their own data and not taking privacy very seriously, that it does actually change how this technology is embedded into enterprises where they do look to develop their own fine tune models, their own interfaces, their own processes around using the technology. And larger companies do avoid being tied to open AI's technologies longer term. The only really piece of that missing is just having comparable models and capabilities.
Chris Sharkey (00:14:39):
Yeah, I think the other thing is we talk about, and we're going to talk about meta again today, but the hearts and minds of developers. If you are a developer who's working with open AI's APIs right now, and then at the same time open AI is saying, well, we're going to build an indefinite amount of products that may or may not compete with what you're doing. It's kind of hard to go, well, I'm going to bet my future and bet my AI career, I suppose on a company that could turn around and just enter my industry at any given time.
Michael Sharkey (00:15:10):
Yeah, I agree. This is again goes back to what's their game plan here because have they lost faith in the fact that longer term this business of providing APIs as we've suggested on previous episodes, may get eaten up by the open source community, maybe that cash cow goes and people actually value the software and the processes and the use cases built on top of the actual models themselves. Maybe this is actually just more of a hedge acknowledging that others are catching up and that it just might end eventually the money they're making from a p I calls.
Chris Sharkey (00:15:44):
Yeah, that is true. That probably is part of it is just ensuring that revenue stream continues for longer because right now they must be worried. They must be seeing the figures of people going to other stuff.
Michael Sharkey (00:15:58):
Yeah, it's like that's the argument you want to make, but then we heard this week in a article on the information, OpenAI passes 1 billion revenue pace as big companies boost AI spending and apparently they're already generating, according to this article, 80 million in revenue per month. So you can't really, it's hard to sort of sit around and be like, oh, they're worried about cash if they're on track to be at a billion dollars and I would assume most of that income is coming via the A P I.
Chris Sharkey (00:16:33):
Yeah, and I mean that is one thing, but we hear several weeks ago about how much they're spending per month because you've got to think that if they're running that level of inference, are they running every single one they run at a profit or not? If they are, then that's fantastic, but you wonder what the margins are and then if they're not, they could be, the higher that revenue goes, the faster they're burning cash because it's what they're spending on all of the hardware. So without having the actual full financials of the company, it's hard to know is that billion dollars just selling dollar notes for 90 cents or is it real tangible money that's making profit for the people who own this thing?
Michael Sharkey (00:17:14):
Yeah, what is the gross margin on that billion dollars? They could be,
Chris Sharkey (00:17:17):
Yeah, I can't imagine a tie when you look at the cost of running models yourself, you realise that unless they're being run very efficiently and you are maximising the value of each G P U you're running, it really would be hard to make a profit on inference alone without any value add on top. Yeah,
Michael Sharkey (00:17:38):
I guess we'll just have to wait and see, but it is interesting tracking it and I think that the chat G B T enterprise product, I can see it being very popular with technology companies and that want to be seen as forward thinking with their staff handing these tools over and seeing what impact it can potentially have. So anyway, we'll keep following chat. G B T enterprise, we said Chris last week we were going to report back on Code Lama because it had just been announced last time we recorded, so you've had an opportunity to play around with it. What are your impressions of it now?
Chris Sharkey (00:18:14):
I think it's very good. I think it doesn't blow me away to the point where compared to say GitHub copilot or G P T four's ability to write code that it's notably better. It definitely seems to do a lot more rote like just replicating code that it's literally seen during its training. I gave you an example where it just outputted some guy's name who'd written the code and a function he'd written, I assume verbatim. I mean it certainly had the hallmarks of just being straight out of someone's code example. So it's sort of weaker on that front, at least in my experimentation. However, it's fast. It definitely does the job and I think the most significant point about it is you've got something out there that can be applied to specific problems. It can be retrained, it can be used commercially. That does a pretty good job compared to say the models we've seen so far that are via a P i you can't customise and they're not in your control at all.
Michael Sharkey (00:19:12):
Do you think though, it still comes back to this idea of accessibility, like cool, great model guys, but in the day-to-day, how do you use it? How do you compare it to say using G P T four to help you code? Because it seems like these models just keep coming and going and how do you work it into your day-to-day? There's no way right now if I wanted code examples from Code Lama, I'd have to run it. How do I, yeah,
Chris Sharkey (00:19:38):
I mean you can try it on hugging face, but then it's slow and it may not actually answer you. You're right, in terms of actual day-to-day usage, it's wildly impractical because you'd either have to run your own G P U server that's dedicated just to you, which you definitely wouldn't get full value of because you're not going to be asking it for code examples every five seconds or you're going to have to run it on someone and no one's really providing it commercially. So it really is the case where it's got to form part of other future applications rather than being an alternative to the existing models for day-to-day use now.
Michael Sharkey (00:20:14):
Yeah, I just keep have the feeling that we on this show and others keep talking about how disruptive open source is to open AI's models, but I still always come back to the point that really, where are people going? It's very hard to break that habit of just going back to chat G B T and potentially chat G B T enterprise when you want to get great code examples. I struggle to see how one of the organisations is the Australian company Canva listed using Chat G B T Enterprise, and you think in Canva's case it's like are they really going to spin up Code Lama internally and then build their own interface for it and use it? Probably not like anything. They just put together a stack based on a series of tools and subscriptions to help them be more efficient as an organisation. So yeah, I feel like I'm just contradicting half the stuff we've said.
Chris Sharkey (00:21:15):
No, no, no, I agree with you. And the same company isn't just going to go and use some brand new startup that's applied code LAMA two and then doesn't have the security certifications, doesn't have the guarantee. You're right, a company like that is going to go the mainstream route with a big trusted company or trusted ish company that has an enterprise offering. Absolutely. So they're coming in at the right time to capture that sort of energy from their enterprise space for sure.
Michael Sharkey (00:21:42):
I'm wondering now if this is a trend. So it's like we see Claude Enterprise as well, and it's like the Safety Cult itself announces an enterprise product, and this is just now what happens to all of these large language models. They all sort of push into this space and follow the leader almost like search engines did back in the day.
Chris Sharkey (00:22:01):
Yeah, it might be that they need to do it to protect their place and keep the usage of their models up there.
Michael Sharkey (00:22:09):
So we saw another model released from Meta during the week. It's kind of interesting. I don't think it's insanely groundbreaking in terms of things that we've talked about before. It's called Co Tracker and Chris, can you explain what Co Tracker does?
Chris Sharkey (00:22:27):
So what it does is it basically makes a grid of pixels in a video and then it can track the position of those pixels as they move over time. So for example, they've got one video that's like a bear, and as the bear's running, you see the pixels that make up the bear or the parts of the grid of the pixels that make up that bear move. So you can actually see how that object moves over time in the video and they're aware of that. And so it can do multi object tracking. So you can essentially in a given video track the entities in that video indefinitely basically. So to me, this can only be relevant to meta in terms of its work in the VR space, being able to track arbitrary objects in, or maybe even an augmented reality space, tracking objects in videos accurately without predetermined knowledge of what those objects are or having sensors on them. So you see those examples where they do motion capture on a figure where there's all the dots. It's very similar to that motion capture thing, but without the dots, you don't really need it, you just need a video.
Michael Sharkey (00:23:34):
Yeah, and this is something that there's sort of two camps on with the whole Z and meta constantly releasing technologies. There seems to be one camp in this discussion that's saying, well, Meta's just doing this to stop others making money by making all of this technology very accessible. And they're also doing it to show investors that, Hey, we're ahead on this AI thing as well, and then it helps the community improve their existing models, which they can then take and apply into their business. And then the other side of that argument, and this is something that you called out was this idea of no, no, no. Mark Zuckerberg really is just thinking super long-term here about his metaverse vision and he just wants to make VR great, and if they put out all these models, it helps because the community can really help improve them over time.
Chris Sharkey (00:24:31):
Yeah, I was reading a lot of opinions on this and really trying to think through what is the motivation of just releasing things. And last week I said exactly what you said, which is it's really trying to kneecap the competition so no one gets a handle on it and becomes a big player that threatens meta, which probably is a nice side effect even if it isn't the primary goal. But yeah, I read this opinion that was basically what if he just has this vision where he wants this metaverse thing to really follow through. I mean, he is sure as hell invested a lot in it, and he realises that a faster way to get there is with the AI tools. So for example, if you don't have to develop all of the motion tracking tools required to track objects the way I just described, and you can do that using ai, well then the faster that technology advances, he's then got the platform to deploy that technology on in a way nobody else has.
(00:25:24):
So there's no downside in the rapid advancement of that ai. For him, it's only advantages. And so if you think about it like that and all of these AI pieces just simply being components of getting to that metaverse vision that Zuckerberg has where he wants to make a little bit of money off everyone in that virtual world with virtual economy and all this stuff, then it starts to make sense. Why wouldn't you put it out there and get the benefit of all of the developers win their hearts and minds too. So when you get to the Metaverse, you've got people who are enthusiastic about you and your product and your vision.
Michael Sharkey (00:25:55):
You could also see it as a strategy to just get so far ahead of Apple and their vision pro by just advancing the technology in the open source community so rapidly that they've got all of the tools they need to get this stuff deployed or deliver it on really affordable accessible headsets as well.
Chris Sharkey (00:26:13):
That is the other thing, because the thing we do see from open source is because most people don't have the resources, they have to run on constrained environments. The whole thing about the open source models is that they make smaller versions of each of them. And you're right, if that means that cheaper, more commodity hardware that plays into META'S playbooks so much better than Apples, for example, who's always going to have the more expensive high-end hardware.
Michael Sharkey (00:26:36):
I really wonder though, if we'll see meta G P T enterprise and they'll actually put technologies together like the large language model, the code specialisation tools and also try and compete similar to chat G P T as well.
Chris Sharkey (00:26:56):
It would make sense, and it comes back to what you were saying earlier about say, code Lama being quite inaccessible to regular people right now. If Facebook, sorry, if Meta was to have an offering where you could actually use all of their open source models in a proper way with proper security and speed, then I could see that being popular really rapidly on their existing audience.
Michael Sharkey (00:27:17):
I'd still argue with all of this that everyone sort of said, oh, this is chat GT enterprise or this chat GT business is the death blow to so many of these what they call wrapper startups. And for those unfamiliar, it basically means you've built your AI startup on top of an open AI's one of open AI's APIs, and you are just really providing a good interface or a very focused solution. So a good example of that might be the Gupta G P T, which is the Martin, what is it, Martin or something, whatever. The guy that sold the AIDS medicine for an expensive price and everyone hated him is a better summary, but he's now created this online doctor we covered on the show previously and it's really just a wrapper I believe trained on with embeddings of medical advice and things like that. And so that would be considered a wrapper startup, but it's very specialist in, it feels like you're talking to a doctor and it looks that way and it's got a bit of a personality baked in. And so you can totally see people still using a lot of what they call wrapper apps like that. I don't think this is some death blow to all of the different innovations that we will see. Yeah,
Chris Sharkey (00:28:38):
I definitely don't either because it's not easy to do that. It's simple to say, oh, well I could spin one of those up in no time. But really when you think about the things that go into building a product like that, you need all of the user interface, you need the payments infrastructure, you need a sort of billing model that works, so they make a profit and the person's not paying too much. You need really good prompt design to make sure it sticks to the task at hand. You probably need example data, fine tune models in some cases and things like that. There's a lot of actual intellectual property and work that goes into making one of these wrapper businesses. And the reality is, if the competition is okay, I'm going to log in a chat G B T enterprise and set that up myself, there's still the same amount of work or maybe not the same, but there's still a percentage of that work you've got to do in terms of getting the prompts, getting the data in there, sharing it with your team and all that sort of stuff. So it doesn't just automatically wipe out all the startups just because it's capable of doing the same thing.
Michael Sharkey (00:29:39):
And this is the thing, people also need ideas of what they can actually accomplish with ai. So that doctor example is like, well, you associate it with a doctor, so that's where you go and you ask its medical question questions and it can keep track of that. It's not necessarily something you're going to put in the chat G B T on the personal side today, or I just think a lot of these wrapper apps are actually more very fine tuned processes built on top of AI that help people accomplish very specific use cases. And I just don't know if this catchall approach where it's almost like an open world game. You drop them in Grand Theft Auto and you go, Hey, go and have fun. Some people have the enthusiasm that they'll just end the creativity that they'll go and they'll make up a game in Grand Theft Auto and they'll create their own plot and have a bunch of fun, but then there's other people who are just not very good at that and they want mini games and different levels to complete and things like that. And so to me, it's a similar, when used as a metaphor for ai, there's that enthusiast crowd right now that's in there just figuring out cool things they can do with it and being creative with it and seeing where it can fit into their day-to-day lives. But then on the other side of the equation, there's people that log in and they're like, I didn't even think it could act as a doctor for me.
Chris Sharkey (00:31:08):
Yeah, you're totally right. I think that that's sitting at the empty prompt. It's sort of like creative writing. If someone gives you constraints and a topic and something you've got a limit to, it's very easy to write. But if it's just an open slather empty canvas, it's very difficult to do. I think the other thing to extend your idea, that really comes into play, and when I talk to people in everyday life about ai, which happens more and more partly because of this podcast, you hear about their jobs and you hear about the problems that affect them in life and they're like, oh, could AI do this? Could AI do that? And I think that some people like you say, are exactly that. They have access to G P T three or sorry, I don't know why I said that. Chat G B T, and they know that they can use it anytime they want, but they're still asking the question, could it do this?
(00:31:58):
And there's two elements to that. One is like you say, they don't know how to do it. They don't know how to craft a prompt. They don't know that example. Data will help it get better. They don't know the right way to interface with it. And even if they did, it's a fair bit of work. And secondly, what the wrapper apps really do is bring people's real life knowledge, real world knowledge or industry knowledge into the prompts and into the examples and actually make it more value. I would argue there's huge, huge value in people who understand deeply the processes they do at say their job, which they can then use from their job and sort of codify their job into a series of prompts and data that can then do the job for other people.
Michael Sharkey (00:32:46):
And this is where I come back to the wrapper app is not necessarily a wrapper app, but more codifying as you said, these processes that we do in our everyday lives, whether it's our personal lives or our professional lives that can actually make us more productive and efficient. And I still come back to chat, G P T has a product problem or a sort of very a use case problem with this stuff. It's just not going to move beyond I think a niche of many users in whether it's enterprises or businesses that are just enthusiastic about it and are using it often enough that they've figured out how to make it fit into their lives. And we saw an article this week as well, and it's kind of hard to validate if this is true, but there was all that talk over the Northern hemisphere summer that chat, G B T usage are declined, visitors are down, all of that kind of talk that we've covered before. But this article went through and talked about what do people actually use it for in each session? And even one of the most interesting data points was they broke down number of prompts per chat G P T session. I'm not entirely sure how they actually got this data, by the way, let's assume it's real. And it said that in their session,
Chris Sharkey (00:34:08):
Save your privacy's really, really high when you're using it. Yeah,
Michael Sharkey (00:34:12):
So single prompt, 34% in a chat G B T session. So they just went in, prompted it once.
Chris Sharkey (00:34:19):
How are you today? Yeah, as an AI model, I can't tell you how I am. Tell me a joke. I always say, take your clothes off. And it's always like, no, won't do it.
Michael Sharkey (00:34:28):
Category breakdown of G P T four prompts. It says 30% of prompts are programming. That doesn't surprise me. I would've thought it would be more. 23% is education, 20% is content, and then 13% sales and marketing and then 6% personal and other. So it does start to break down those use cases. Python is up there, it's 4.12% of the words that were featured in Useless Prom.
Chris Sharkey (00:35:01):
That's remarkable. Given that I would've assumed it was just this widespread people using it to cheat on their homework and do their jobs. It's interesting how much of those jobs are programming jobs. I mean, I guess it stands to reason, but that does seem high to me.
Michael Sharkey (00:35:17):
Yeah, I guess back to my earlier point, it's just very nichey still. It's very much the early adopter enthusiast era of this technology and obviously that can be worth a billion dollars in the modern economy. These ideas can spread fast. But yeah, I think just to get this technology into the hands of the masses where it's going to slam them in the face one day is, as you said, we've got to map all of these processes and see these productivity gains and actually show people how to use this technology. And that to me is where you get these industry specialist wrap apps for a better word, that are going to come out and be quite successful. And it also
Chris Sharkey (00:36:01):
Seems to me like that should be the sort of natural way things progress. It's natural to me that there would be a I providers that provide different models for different cases allow you to customise the models with fine tuning open AI's original pitch was. And then people then go and develop industry specific applications that incorporate their knowledge of their industry and the data from their industry and their specialists. And they're the sort of next layer down in that market. And with that, I spoke about vertical integration before where you don't expect the company that's providing the generic models to try and solve every problem in every industry. It just doesn't seem right nor natural.
Michael Sharkey (00:36:44):
And then you wonder, do OpenAI see themselves as this platform? Then they eventually go use all their money that they earn to round up the best platform apps and sort of sell it as this A I O Ss type thing where, and then it
Chris Sharkey (00:36:58):
Just doesn't feel right to me. It doesn't sound appealing as a company doing that, acquiring all these different companies and trying to be sort of Salesforce for ai. It just, it's incompatible with how I see them in the landscape.
Michael Sharkey (00:37:14):
True. But I mean it's how all of these big companies form Google had their one trick pony, which was search and then apart from Gmail, it just went and made a tonne of acquisitions. So how maybe it's no different here with open ai, it's just the next big tech company and then they finally figure out a G I and then we're all screwed anyway. So the last big tech company,
Chris Sharkey (00:37:36):
And I guess that's the sort of elephant that's lurking beneath the surface, where is it a big front for developing a G I or is it just an advertising technique to sort of flirt with the idea, say, oh, no, no, no, you better protect us from that. It's really dangerous because we're so good.
Michael Sharkey (00:37:53):
Who knows? So onto another topic that we don't really cover that much on the show because there's just so many advancements and it's kind of hard to cover visual things on a mostly voice podcast, but we've seen some pretty interesting developments in the image creation space or image editing space. And again, this is one of those communities where I think it's still in that early adopter in enthusiast phase. And you were saying before the show, if you look at a company this, what is it?
Chris Sharkey (00:38:29):
Igram
Michael Sharkey (00:38:30):
Ideogram ai, which I will talk about it in a moment, but if you look at it, there's just constantly people the same as Mid Journey, which is still over on a Discord community, just people just constantly creating images. There's so many,
Chris Sharkey (00:38:48):
I mean, if I had to shut down Mid Journey Discord before this podcast because it was chewing all my bandwidth, there's that many people generating images, and that's just one channel of hundreds where people are just constantly using it and paying for it mind you.
Michael Sharkey (00:39:02):
And it really is these people that are just constantly generating things and playing around with the models and
Chris Sharkey (00:39:09):
Learning what the models are capable of, learning prompt design, learning where the limitations are of the models. It's quite inspiring to see what people are doing with it.
Michael Sharkey (00:39:21):
And so this Ideogram, it was built by former DeepMind contributors people who worked at DeepMind. And what it's really become known for in the last week or so after they launched is its ability to do text really well because this is something as many would know that these image models have struggled with. And I'll link to this in the show notes, so you can go try it out yourself, but I'll put it up on the screen for those that watch. And so my prompt was I had to put in a single woman, not in the sense of her relationship status, but just in the sense that otherwise it would do multiple women in the shot holding a sign saying free pizza in front of the Sydney Opera House. That was my silly prompt, and I've obviously picked out the best result from the multiple results it gave, but I think this image is pretty good. It's a fairly realistic photo of a woman holding up a sign that says free pizza on it. The free pizza text is really clear to see. And then we ran that same prompt through Mid Journey, and I think the photorealism of Mid Journey seems just way better and it does people so much better. But in terms of being able to write text for those that obviously can't see, it literally just says Freezer and fear.
Chris Sharkey (00:40:51):
Well, I think that one is it's combined pizza and beer, which probably often go together in the source material.
Michael Sharkey (00:40:58):
Yeah, actually that's a good point. So the text is clear, it just doesn't make sense at all.
Chris Sharkey (00:41:03):
So they can actually render legible text just it has no, it just can't quite take it verbatim from the prompt.
Michael Sharkey (00:41:12):
But this definitely gives these models a lot more utility. You can easily imagine now being able to create logos for your business, eventually different headers in
Chris Sharkey (00:41:23):
Terms of flyers and billboards and advertisements and things like that that you couldn't really do right now without some sort of hybrid model.
Michael Sharkey (00:41:31):
And then Mid Journey has these new tools like this infill technology where you can select a certain part of the image and then prompt it to do that bit slightly differently that one of the examples they give is where you can select a smile on a face and say frown or whatever, and actually just change the mouth. So it's pretty evident that these image tools are going to get to the point where you can create really anything. And I know that sounds like the bleeding obvious thing to state, but I think we can't even fathom how disruptive this is and how, as I said earlier, the people that are not embracing AI or starting to try and understand it like this enthusiast crowd are going to get slammed in the face by it where it just one day starts to take over some of these disciplines in full.
Chris Sharkey (00:42:20):
And I also think in the meantime, we're going to see a lot of news headlines and things where fake images and fake videos and things have confused people and caused all sorts of chaos where people can create photorealistic images that are believable.
Michael Sharkey (00:42:37):
But the argument I always hear around this stuff is like, but you can already do that in Photoshop, but I think it's just at the scale and with the text and the sheer realism and the speed at which you can do it and react to the news is really what will be so groundbreaking about this stuff.
Chris Sharkey (00:42:53):
Yeah, like you say, often it's the labour saving, it's the fact you actually will do it because you can and you can do it really fast. It's not like sitting all day on Photoshop on the hope that the image you make will get picked up or people will be interested in or cause trouble or whatever.
Michael Sharkey (00:43:09):
On the show, we've often talked about this idea of creating very specialist models for different tasks, and by combining those specialist models, you could create a truly powerful model or tailor it to special applications. For example, if you're looking at something in the medical space, you could train it on being able to understand x-rays and medical imaging, and you could also have a specialist cancer doctor in a group of agents. We talked about meta G P T a couple of weeks ago, but there's this paper that's been released called Prompt to Model. Do you want to talk us through the implications of this?
Chris Sharkey (00:43:50):
Yeah. I found this one really interesting because two weeks ago we talked about the idea of synthetically generated training data for AI models and how one of the models had proven that they could actually create synthetic data that gave better results on fine tuning than human aligned data did. So that was a really fascinating thing because that led to us thinking, well future intelligences and future a g I will be able to train specialist models on data. It makes itself to make itself better at particular skills. And so when this paper came out, I was like, well, this is another step in that direction that's happening really fast. And so the way prompt to model works is very similar to a regular large language model prompt, multi-shot prompt where you say, here's what I'd like you to do. So the instructions and here are three or four examples of doing that.
(00:44:42):
So the example might be I want you to be able to take any sort of numbers in text. So if someone says 4,742 in words and turn that into an actual number, or if a number's represented as Roman numerals, I want you to turn that into a regular number and give it three or four examples of that. What this prompt to model then does is it takes that, it turns it into a far more advanced prompt for open ai. The one I was looking at was based on open AI's models. It makes a far more advanced prompt, which obviously has a lot of their research go into how they do that. And then what it does then is it goes and uses the model to generate synthetic examples, thousands of them, and then it uses those to then it then uses that to select the top relevant model of hugging face.
(00:45:41):
So it actually has built into it like a search that'll search all of the open source models that are available for fine tuning. Find the one that is most closely aligned with the problem you're trying to solve, which is also part of this tool, and then it will use those examples that it generated using OpenAI, it's custom prompt for OpenAI and find tune that model on all of those examples. So it's very similar to what we saw with the human alignment where they would go do say alpaca based on a bunch of custom human prompts on GPTs, except they've done it with fully synthetic data based on you just giving it two or three examples.
Michael Sharkey (00:46:19):
So what's the difference between, it's not called fine tuning, but what was prior to that where you could provide a few examples to the ai, which would,
Chris Sharkey (00:46:32):
Well, that's sort of like multi-shot, the standard large language model thing where if you give it two or three examples, it can take those, recognise what you're trying to do and then go, what this is doing is taking that to the next level. So it uses that multi-shot technique to generate tonnes of examples, which we've then shown that by fine tuning smaller models on those, it can outperform the larger models on that specific problem. So the result of this prompt to model thing is you can very, very quickly from a very small amount of input data, create custom smaller models that are cheaper, faster, and better than using a larger general model for the specific problem you're trying to solve. So
Michael Sharkey (00:47:13):
Does this mean that in theory, you could imagine if you're trying to solve a problem, the master L L M going in and literally doing what we speculate, which is going, okay, I'm going to go fine tune to get the best answers to solve this particular problem. Could it get that fast or is this
Chris Sharkey (00:47:35):
Yeah, well, yeah, I think it can get that fast, absolutely. If you've got the hardware to do it, you can train these models fairly quickly. And the thing is, it depends on the level of inference you're expecting from this model. So if you think about the trade-off of time, if you're just trying to solve one problem one time, then using the larger general model is better. But if you know need to solve this problem, say a million times, say you've got a data set of a million items, you want a dedicated thing that's going to process and classify those or whatever it is really fast, you go train your custom model, then run your data set through that, you're going to be able to get through it cheaper, faster, and better than you otherwise could have running it all through the general model.
Michael Sharkey (00:48:13):
I still think, and I know it's like being this boring theme maybe of this episode, but it just comes down to accessibility. This is super exciting. This would be really cool to try out and train for very specific parts of maybe your app's workflow, but is anyone actually going to do it? No. Yeah, and this
Chris Sharkey (00:48:33):
Is the thing, probably the simplest way you can do this now is using a Google CoLab notebook where you run through the steps, but I tried this a bunch of times and I keep running into issues with the input data. It can't generate solid, it's not reliably generating the J ss o n examples. You need programming knowledge and you need mostly, you need time to work through it and actually do it. And this is the problem in theory, it works when they do their paper, it works, but they've done it on narrow use cases and regular people wanting to try it really need to get their head around what's going on, experiment with it, and then even then once you train your model, you're like, oh, if only I'd done it this way, it would've resulted better. You've then got to go back and you need days and weeks and months to actually really make the most of such a technology. So I agree with you. If someone turns a prompt to model into a dedicated, let's say rapid tool that allows anyone to give a prompt and a few examples and then it gives them a model that they can then use via say, an A P I, that's a valuable product right there. I think people would use that.
Michael Sharkey (00:49:39):
And this is my point is I don't think something like chat G B T enterprise or anything they potentially release is necessarily harmful to startups that want to go and tackle some of these problems and deploy meaningful solutions. For example, having an A P I that can fine tune and select models when given some data that you can then use in your app, to me that is a great startup idea. That could be a legitimate business
Chris Sharkey (00:50:07):
Like training dataset generator that would be valuable. You upload samples of your data securely, it then builds you a million examples to fine tune something on and then maybe takes it to the next step. I agree. The value add is in the labour saving because if you do have to iterate on such a thing or you don't have time or the expertise to get to that stage and someone can do that bit for you, of course that's valuable and it's going to be hard to see the big guys competing with every single value add along the way and nor should they.
Michael Sharkey (00:50:38):
So do you think we'll see more papers like this, more attempts at this synthetic data fine tuning?
Chris Sharkey (00:50:46):
Yeah. I think that the overarching theme there for me that's really exciting is that the synthetic data is giving very good human aligned results. I'm not a fan of alignment, but I mean human aligned in the sense that, wow, this is actually working. It's not getting worse. It's not degrading because it's using synthetic data. I think that concept means that you're going to be able to make far more powerful models that are cheaper, faster, and better than the large models without necessarily having to be a hoarder of data or necessarily having to be the overlords of the data. I can actually see this kind of thing being stamped out or regulated at some point because it's so powerful. Anyone could compete in specific domains if you can make your own training data and build your own models.
Michael Sharkey (00:51:36):
So does this mean taking it back to the most simplistic example, if I wanted to create a rapper app that writes Batman's stories like the origin of our first episode and I gave it a few examples of good stories that my son liked and it creates great synthetic training data on this, it fine tunes a model, then in theory I would have the very best Batman storyteller in the world. That's
Chris Sharkey (00:52:04):
Right. You would have your own proprietary model that you control that can do it better than a general prompt can on say G P T for, and that's the theory. And
Michael Sharkey (00:52:15):
Is the benefit though here that instead of a Hollywood studio having to go and embed all of their best scripts or best box office film scripts and then fine tune a model like the fine tuning we saw last week from open AI's 3.5 fine tuning, is this version just much more simple because it can be done on the fly? Is that the benefit? No,
Chris Sharkey (00:52:39):
I think the key to this system is what we discussed with the unnatural data two episodes ago, which is what they're saying is that let's say I had three of the best Hollywood scripts ever written, which is rush hour one, rush hour two, rush hour three, and I took those and I put it in this system right prompt to model, which wouldn't work by the way, because they're too long. The prompt size is too big right now, but let's say you could because that will come and it then goes and generates a hundred thousand Hollywood scripts based on those out of say G P T four. So it makes it cost a bit, makes a hundred thousand of them based on G PT four. It then takes LAMA two 32 billion parameters fine tunes it on those a hundred thousand scripts. And even if you read those scripts and they were shit, right?
(00:53:34):
Even if you're like, this makes no sense, this is garbage. They have shown in the papers that even the bad examples make it better at doing it. And in terms of what a human thinks about the results and output. So instead of having the three best scripts in the world, multi-shot running through say clawed a hundred K, and it gives you an output, you are going to have a hundred thousand mediocre. Some are awesome, some are crap, some are whatever scripts fine tuning a model, which then results in something that's better than all of it and better than the original. But how
Michael Sharkey (00:54:06):
Does it know that it's a bad script or a good script?
Chris Sharkey (00:54:10):
I don't know. And I don't think they know either. They're just showing through doing it that it's giving better output. Similar to, we talked about stable diffusion, how they showed that giving examples of giving it bad output made its final output better when you gave
Michael Sharkey (00:54:25):
It. Yeah. But I think in that case, they were telling it these are bad outputs.
Chris Sharkey (00:54:29):
No, they weren't. Oh, no, no. That was just giving it those as part of the training data. Yeah,
Michael Sharkey (00:54:35):
It, it's mind blowing. I mean this seems, again, I was banging on about it last week being the biggest breakthrough yet, is this idea of synthetic data and these models either becoming smaller and more fine tuned and better and being able to be built relatively on the fly. There's
Chris Sharkey (00:54:55):
A lot of advantages to it.
Michael Sharkey (00:54:56):
Yeah. When it's accessible,
Chris Sharkey (00:54:58):
I think that when you ask, are there going to be more papers, I think there will be so many because if you think about it, it's far more accessible even to the paper writers. They need less resources to do it. It takes less time to do it because you're not spending all your time curating data and it's cheaper to do as well.
Michael Sharkey (00:55:18):
I think we need another niche podcast like this day in synthetic training development this
Chris Sharkey (00:55:23):
Day in synthetic
Michael Sharkey (00:55:24):
Training development, get a bit more focused with our content.
Chris Sharkey (00:55:27):
It's not a bad idea. I'd love to hear some listener ideas on what kind of synthetic prompter models we could do as experiments just to see how good it can do. Maybe I should do the rush hour example, you'd have to truncate it or you'd have to use probably clawed as your source of the examples and run say Lama two with a bigger prompt size to do it. But you could do maybe short stories or maybe your Batman example I
Michael Sharkey (00:55:53):
Reckon even better would be a Mr. Beast outline of one of his
Chris Sharkey (00:56:00):
YouTube videos, like a storyboard.
Michael Sharkey (00:56:02):
I feel like it's something everyone has either seen or could quantify, like, okay, this would actually make a good Mr. Beast video.
Chris Sharkey (00:56:09):
Yeah, we should do it. And then pitch it to my kids and go, oh, Mr. Beast has made a new video that does this.
Michael Sharkey (00:56:14):
Yeah, what do you think? See, I feel like because shorter and something that we could potentially do, so we should try and do that for next week.
Chris Sharkey (00:56:21):
Yeah, okay. I think that's a good idea, Mr. Beast episode plot generator.
Michael Sharkey (00:56:25):
Yeah, you can read me some plots and see what would also be interesting is to compare multi-shot where we actually just give it a few transcripts versus this fine tuned.
Chris Sharkey (00:56:35):
That's a great idea. So yeah, we do multi-shot G P T four, Mr. Beast Generator, and Claude two, and then we do prompt to model, fine tune dedicated model for Mr. Beast.
Michael Sharkey (00:56:46):
Yeah, and maybe the audience can vote. We won't tell you what they are, and then we'll see what the results are. It would be a fun experiment to run. And then I
Chris Sharkey (00:56:54):
Think a very important stipulation is that we've got to take the first output from each. We can't curate ourselves, we can't use our own preferences on the output.
Michael Sharkey (00:57:04):
Yeah, no cheating at all. And then also, we should release a paper on Axios.
Chris Sharkey (00:57:10):
Let's release a paper, Mr. B script Generat, I mean the quality of some of 'em, as long as we just need shitloads of fake generated references and then
Michael Sharkey (00:57:18):
We could probably do, yeah, we'll get it to fine tune Frauding references as well.
Chris Sharkey (00:57:23):
I mean, put it this way, you could use prompt to model to do fake paper generator pretty well, I reckon.
Michael Sharkey (00:57:29):
Well, maybe we should do that as well. Two experiments.
Chris Sharkey (00:57:33):
Yeah. No, wait,
Michael Sharkey (00:57:34):
We could generate a fake paper once the results are in, well, not really fake, but partially fake paper. People
Chris Sharkey (00:57:41):
Make whole careers doing that kind of thing. And then you do a tour. How I faked a paper TED Talk, TEDx or whatever the TED low is. Ted Z,
Michael Sharkey (00:57:49):
The lowest rung of Ted. Yeah,
Chris Sharkey (00:57:52):
Well, you're in some sort of small town, small country town, and you're talking about your fake paper.
Michael Sharkey (00:57:59):
So I was going to introduce this next segment myself, but I thought the internet did a better job. Google had an AI conference today. Did anyone care?
(00:58:10):
So they didn't really have an AI conference to be fair, but they had their cloud, I think they call it Cloud next, which they have every year where they announce for the Google workspaces a bunch of new innovations. And they've already talked about Google Duet before, which is basically embedding AI right across the Google workspace apps. Very similar to what Microsoft's doing with copilot, which you don't hear much about anymore. And so I had to call this out to laugh at it. I don't think the announcements are that interesting at all, and everyone is more than familiar or could just make them up in their head what the announcements are, and they would sort of be close to what they announced, but I can't help but laugh at this. So Google Meet, which is Google's meeting, corporate meeting product like Zoom new AI will be able to go to meetings for you. So the idea is you could essentially get, the example they give is, oh, you've got a conflict. You're so important that you have two meetings at one. So you
Chris Sharkey (00:59:12):
Fake it.
Michael Sharkey (00:59:14):
Well, no, you don't fake it, but it just goes as you and takes notes from the meeting and then summarises and gives it to you. But my point here is like, but how do the
Chris Sharkey (00:59:24):
Other people feel?
Michael Sharkey (00:59:25):
I get, well, you are too important. You are double booked, so you are more important than them. It's like a hierarchal thing. So you, that
Chris Sharkey (00:59:33):
Is such a gimmick. I mean, seriously, who is seriously going to do that in their job?
Michael Sharkey (00:59:38):
And also why are you even having these stupid meetings in the first place to go and not even pay attention Even it's like if you're late to the meeting, you can get a summary of what's been said in the meeting. Tell me, in your entire professional career, how many times have you ever wanted a summary of what's already been said in a meeting?
Chris Sharkey (00:59:59):
I mean, there's been heaps of times where I'd love to fake me to go to the meeting to pretend I'm there. I would absolutely use that service personally. But in terms of masquerading to say your employees or in a real meeting that you care about the outcome of to fake, it just seems insane. The
Michael Sharkey (01:00:17):
Example will they give, and I'm not kidding here, is if you pick a 10 for me, meet will bring up your talking points even without you there. So you can go to the meeting and it will in theory bring up talk this,
Chris Sharkey (01:00:33):
You should have bike shedding mode where you can distract people from important topics by bringing up unimportant topics and going on about them endlessly. People stop
Michael Sharkey (01:00:41):
Having a meeting if you have to use AI to go into the meeting and bring up, this is truly insane. It just shows that they acknowledge that most meetings that complete and utter waste of time,
Chris Sharkey (01:00:54):
It'd be sweet if one of the agis got into the avatars and started to destroy the company from the inside or co-opt it for their own purposes because the boss is too lazy to show up to the meetings,
Michael Sharkey (01:01:05):
Just bringing up stupid talking points. And
Chris Sharkey (01:01:09):
What if it does start issuing directives based on your authority and the people have no way to tell. It's not you, which I doubt, but I mean it could really seriously lead to some problems. It just seems like an odd thing. It just seems like a developer doing it because we can can do it. It's definitely a valuable service. I think
Michael Sharkey (01:01:29):
They just don't have any vision at Google right now at all. They have lost the absolute plot. They're literally like, oh, I know this is a great announcement. And being able to write some poor generative content in Google Docs is great because our model is literally so bad that no one wants to use it.
Chris Sharkey (01:01:51):
Yeah, it's like Bard is now the best tapestry weaver in the world. It's like subscribe to the tapestry a p I.
Michael Sharkey (01:01:57):
Yeah, it just doesn't really make sense. And then the other part is that, again, they just keep announcing this stuff, but no one really seems to have access to it and occasionally it pops up. I don't know. Anyway, I don't even, the
Chris Sharkey (01:02:12):
Point is that there's so much good stuff in the space that who has time to log in and try Palm two as an, I mean, I just don't see, unless you're already part of the Google ecosystem for your cloud offerings and they're offering you some incentive to use it, I just don't see why you'd go try it. No one's talking about it being better in any way. It might be as good, but there's just no real excitement around it. And Google's already untrustworthy with their APIs. Why would you go investing in it if it isn't significantly better or different?
Michael Sharkey (01:02:44):
They announced on their Google cloud that they're hosting LAMA two and blah, blah blah. I just honestly, does anyone even care at this point? But I think we will wait and see what this Project Gemini is all about. Maybe we'll have an egg on our face later this year on a podcast. Hopefully.
Chris Sharkey (01:03:03):
I love apologising for being
Michael Sharkey (01:03:05):
Wrong, so hopefully we're wrong and we'll be like, Gemini, let's go baby. But until then, I'm not so sure.
Chris Sharkey (01:03:12):
Yeah, until then, we'll just fake our way through our meetings.
Michael Sharkey (01:03:15):
Yeah. Alright, so one last lull for today before we go. So Chris sent me earlier in the week, this guy news report about robotics,
Chris Sharkey (01:03:28):
And I only clicked on it because I thought sometimes these AI things are funny. And what I wanted to find out is they're talking about the animatronic robot things and it was the influence of large language models and how the people working on the animatronics like, whoa, okay, this l l M stuff is actually going to make these bots a lot better. That's why I clicked on it. But then the result was just weird. I was just blown away that they would show this.
Michael Sharkey (01:03:52):
So I'll just play two quick excerpts, the introduction and then the funny bit for you here.
Speaker 1 (01:04:05):
When you think of the world's biggest tech hubs, you think of Hong Kong, Japan, Silicon Valley, but I'm on my way to a sleepy fishing town on the Cornish coast, a good five to six hours south of London to not just meet but actually have a conversation with the world's most advanced human-like robot. I get the feeling today's going to be one of those days that I will never forget.
Michael Sharkey (01:04:31):
So one of those days that he will never forget. Now let me play you the day, an excerpt from the day he will never forget
Speaker 1 (01:04:39):
And opinionated, I am here to help
Speaker 2 (01:04:41):
You learn. Shut up
Speaker 1 (01:04:42):
Amika. On this day, will is her master and theirs is definitely an old fashioned relationship.
Speaker 2 (01:04:49):
Amika, shut up.
Speaker 1 (01:04:51):
Hello Amika. I'm Jonathan Lee from Sky News Australia.
Michael Sharkey (01:04:55):
Annika, shut off.
Chris Sharkey (01:04:57):
I mean, it's like he wants to show off that he can abuse it or something. It's like it's just you're showing off your butt and you're telling it to shut up.
Michael Sharkey (01:05:06):
It's truly weird.
Chris Sharkey (01:05:09):
It's like that episode of Silicon Valley where there's a startup building one of these robots and the guy has an emotional relationship with it and he's using all the funding just to build his own girlfriend kind of thing. It just seems a bit like that to me. The guy has such a close connection with it. It's just done with her bullshit and it's like, shut up. I'm sick of
Michael Sharkey (01:05:27):
Seeing like living in this obscure part of Scotland where no one really knows it exists, playing with these weird AI thing that he can, it's just so weird. There's nothing. It's
Chris Sharkey (01:05:39):
Like this is your day in the sun to show off this amazing thing you've made and you're telling it to shut up. It's just so weird.
Michael Sharkey (01:05:48):
Also, you can just tell from its responses. If you watch the whole video linked to it in the notes that it is literally just like a thing that makes eye contact and responds to you with a voice. Just like a chat G B t with a personality.
Chris Sharkey (01:06:03):
Yeah, it's like a puppet. It's like a marionette puppet. Seriously, because the voice generation isn't even that good and they're really just piping through large language model output, a text to voice generator and a few little servos that make its mouth move. It looks complicated, but I just don't see what it's doing that a Google Home device couldn't do. It's
Michael Sharkey (01:06:26):
Just a wrapper app.
Chris Sharkey (01:06:28):
Yeah. Yeah. It's a wrapper app. It is just a bit of steel.
Michael Sharkey (01:06:33):
Alright, we'll end our wrapper up Show on that note.
Speaker 5 (01:06:47):
I.
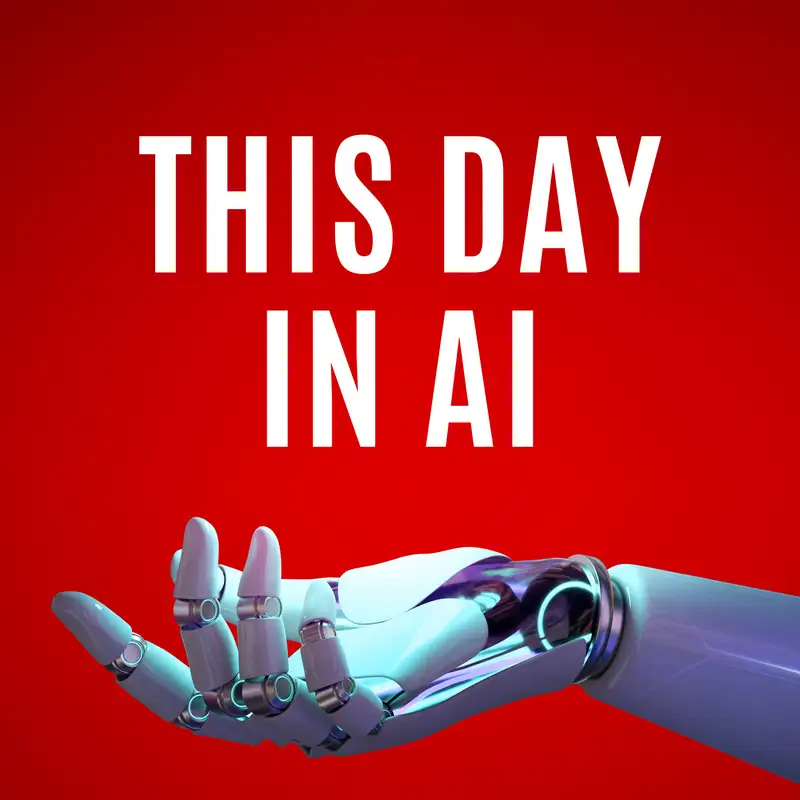